AI-Powered Predictive Analytics: Key Marketing Insights
Explore how AI-powered predictive analytics is reshaping marketing strategies through enhanced customer insights and improved campaign performance.
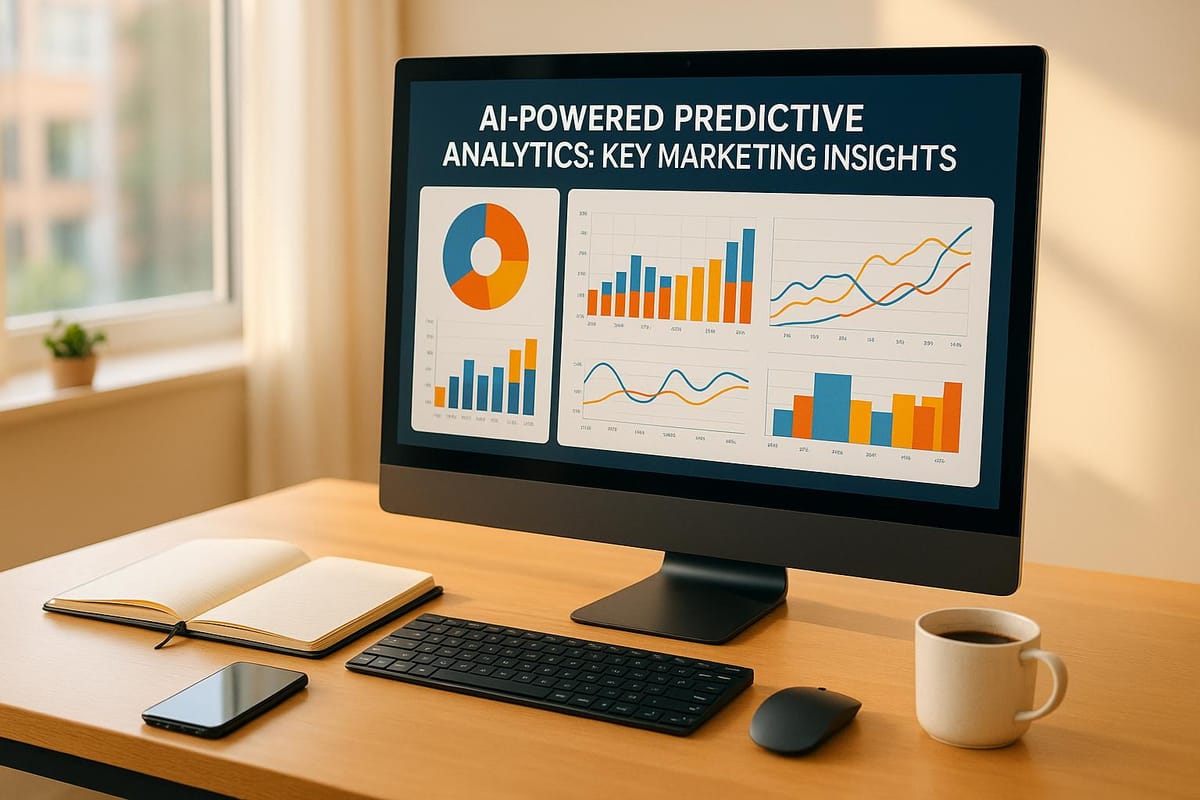
AI-powered predictive analytics is transforming marketing by using machine learning and massive datasets to predict customer behavior, improve campaign performance, and cut costs. Here's what you need to know:
- 91% of marketers are committed to predictive strategies, driving 22.66% conversion increases and influencing 26.34% of orders.
- Machine learning models like neural networks and decision trees are key tools, helping brands like Adidas and Walgreens boost conversion rates by up to 276%.
- Advanced data systems (like data lakes) ensure accurate insights, with companies 23x more likely to gain customers when managing data effectively.
- Predictive analytics enables smarter customer segmentation, personalized campaigns, and up to 20% ROI improvements.
Quick Tip: Combining AI with your marketing tools can cut acquisition costs by 50% while improving efficiency. Ethical practices, transparency, and bias audits are critical to maintaining customer trust.
AI in marketing is no longer optional - it's essential for staying competitive and delivering real-time, data-driven results.
The Rise of Predictive Analytics in Marketing Strategy
Main Elements of AI Predictive Analytics
AI predictive analytics in marketing is built on two key components: machine learning (ML) models and data systems. Research reveals that 75% of marketing organizations have already implemented AI or are actively testing it [3].
Marketing ML Models Explained
Machine learning models are the backbone of AI-driven marketing strategies. For example, a major Taiwanese media outlet achieved a sixfold increase in click-through rates (CTR) and reduced bounce rates by 30% by leveraging ML-powered personalization [3].
ML Model Type | Marketing Use | Key Benefit |
---|---|---|
Neural Networks | Customer Intent Prediction | Recognizes deep patterns in complex customer behavior |
Decision Trees | Customer Value Calculation | Provides clear, transparent decision paths for segmentation |
Random Forests | Campaign Performance Prediction | Increases accuracy by combining multiple decision trees |
Support Vector Machines | Market Trend Analysis | Classifies market segments with precision |
While these models can deliver impressive results, they rely on a strong data infrastructure to function effectively.
Data Systems Requirements
Organizations that excel in managing data are 23 times more likely to acquire new customers and six times more likely to retain existing ones [5]. To achieve this, businesses need to focus on three essential elements:
-
Data Storage Solutions
Advanced storage systems are crucial for handling both structured and unstructured data. Many companies are turning to data lakes and lakehouses for their flexibility and scalability [6]. -
Integration Infrastructure
Seamless integration of data from various sources is critical. For instance, Salesforce Marketing Intelligence streamlined data from hundreds of ad sources, managing over 100 million data rows. This reduced setup time from weeks to just five minutes [Salesforce Engineering, 2024]. -
Data Quality Systems
High-quality data is non-negotiable. Implementing rigorous protocols to eliminate errors and outliers ensures the reliability of AI models.
"AI-ready data means that your data must be representative of the use case, of every pattern, errors, outliers and unexpected emergence that is needed to train or run an AI model for a specific use."
– Gartner [4]
A great example of these principles in action is Walgreens. By using AI-powered ad personalization that incorporated weather and location data, the company saw a 276% increase in CTR and a 64% drop in cost per click [3].
Using Predictive Analytics in Marketing
With the power of advanced data systems and machine learning (ML) models, marketers are using predictive analytics to reshape their strategies. The result? Campaigns that can deliver up to a 20% increase in ROI when segmented effectively [8].
Smarter Customer Segmentation
Predictive analytics has moved beyond simple demographic groupings. It now integrates data from multiple sources - like social media activity, purchase history, and unstructured customer interactions - to create more refined customer profiles [8]. This deeper understanding paves the way for highly personalized campaigns.
Segmentation Type | Data Sources | Impact |
---|---|---|
Behavioral | Website visits, email responses | Identifies high-value prospects |
Predictive Intent | Purchase history, browse patterns | Predicts future purchases |
Engagement Level | Social media, support interactions | Assesses loyalty potential |
This advanced segmentation doesn't just identify potential customers - it drives real results. For example, United Colors of Benetton used a three-stage web push notification strategy to target cart abandoners. Their personalized approach led to a 4.8% conversion rate, a figure seven times higher than the industry average [1].
Boosting Campaign Performance
Once customer groups are clearly defined, tailored campaigns can deliver exceptional outcomes. Personalized, data-driven strategies consistently outperform generic approaches. In fact, companies that excel in personalization generate 40% more revenue than their competitors [2].
Here’s how top brands have used predictive analytics to achieve measurable results:
- Adidas saw a 259% boost in Average Order Value and a 13% increase in conversion rates - all within a single month [1].
- Pierre Cardin leveraged Predictive Ad Audiences (PAA) to achieve a 445% jump in conversion rates and a 164.83% rise in return on ad spend [1].
Cutting Customer Acquisition Costs
Predictive analytics doesn’t just improve campaign performance - it also slashes customer acquisition costs (CAC) while maintaining effectiveness. A few success stories highlight this:
- A fintech company reduced its CAC by 50% in just six months by targeting users interacting with educational content [10].
- A travel agency used predictive insights to optimize Google Ads, cutting CAC by 50% and increasing bookings by 150% [10].
- McDonald’s enhanced social media engagement by 30% and boosted customer retention by 10% within a single quarter [9].
To achieve similar results, companies should focus on:
- Unifying data: Combine CRM systems, web analytics, and survey data to map the entire customer journey.
- Experimenting: Test various ad creatives and adjust based on performance metrics.
- Collaborating across teams: Align marketing and data science teams to refine targeting strategies and improve efficiency [10].
Setting Up AI Predictive Analytics: Step-by-Step Guide
When implemented effectively, AI can deliver impressive results. Take Volkswagen, for example: they saw a 14% increase in dealership orders after using AI-driven ad spend forecasting [12].
Product Feature Analysis
To turn technical features into benefits that resonate with your market, you need a data-driven strategy. A solid data foundation is key to predicting customer behavior and market trends.
Implementation Phase | Key Requirements | Expected Outcomes |
---|---|---|
Data Infrastructure | Clean, structured data warehousing | Accurate feature analysis |
Model Training | Historical customer data, market signals | Predictive customer insights |
Integration | API connections, automation tools | Seamless workflow adoption |
-
Data Quality Assessment
Start by cleaning and organizing your data. High-quality, well-structured data is the backbone of effective AI analysis and personalization [14]. -
Feature Mapping
Pinpoint areas where AI can make a measurable impact. For example, during the pandemic, Levi's used AI to fine-tune pricing strategies while competitors were heavily discounting [12].
Next, we’ll explore how to integrate AI into your marketing tools for maximum impact.
Connecting AI with Marketing Tools
Once you’ve analyzed your product features, the next step is to connect AI with your marketing platforms. This integration can lead to game-changing results. For instance, a B2B software company boosted its conversion rates by 35% by combining predictive lead scoring with its marketing automation system [7].
"We want to use AI to augment the abilities of people, to enable us to accomplish more and to allow us to spend more time on our creative endeavors." – Jeff Dean, Google Senior Fellow [12]
Here’s how to get started:
-
Platform Assessment
Review your current marketing tools and identify where AI can be integrated [13]. -
Pilot Testing
Begin with small-scale projects to test the waters. For example, Monos, a luggage brand, used Meta's machine-learning tools and reduced incremental cost per purchase by 58% compared to their regular campaigns [12]. -
Team Training
Equip your team with both technical skills and creative applications of AI through training sessions and workshops [11]. This is a vital step - only 30% of organizations currently have an AI strategy in place [14].
Keep a close eye on performance metrics. A great example of success is Burger King's "Whopper Detour" campaign, which used location-based AI to drive 1.5 million app downloads and hit the top of the Apple Store charts [12].
When budgeting for AI, consider that custom solutions can range from $6,000 to $300,000 in 2024 [14]. Choose tools that align with your marketing challenges and have the potential to scale alongside your business [11].
Ethics and Rules in AI Marketing
A recent study reveals that 63% of consumers expect clear disclosure when content is AI-generated [15]. This highlights the growing importance of ethics and compliance in AI-driven marketing strategies.
AI Transparency Standards
Transparency in AI marketing goes beyond simply meeting regulations - it's a key factor in establishing and maintaining trust. Businesses need to document their AI models thoroughly and implement strong bias detection systems. According to McKinsey, 50% of consumers are more likely to trust companies that only collect relevant data [16].
Transparency Requirement | Implementation Strategy | Expected Outcome |
---|---|---|
Data Collection | Use clear consent mechanisms | Build user trust |
Algorithm Decisions | Provide explainable AI documentation | Meet regulatory standards |
User Control | Offer opt-out options | Boost customer engagement |
Microsoft stands out as a leader in AI transparency. Their annual AI transparency report reveals that 99% of employees complete responsible AI training, setting a high bar for corporate accountability [17].
"To safeguard the public, governments need to take seriously a wide range of possible scenarios and adopt regulatory frameworks at national and international levels. Regulations should always prioritize public safety."
- Yoshua Bengio, Founder, Mila-Quebec Artificial Intelligence Institute [17]
On the flip side, companies like Clearview AI have faced severe consequences for non-compliance. For instance, Dutch authorities imposed a $34 million fine on Clearview AI for GDPR violations, emphasizing the importance of handling data responsibly. These examples underline how transparency can be a cornerstone of customer trust.
Customer Trust in AI Marketing
Transparency is just one piece of the puzzle - earning customer trust also requires balancing personalization with privacy. Research shows that 40% of companies using AI have experienced unintended bias [19]. Addressing these biases is critical to maintaining credibility.
Take Tapestry, for instance. Its Tell Rexy AI system incorporates ongoing human feedback to ensure the brand's values remain intact. Similarly, Dove’s "AI and Real Beauty" campaign tackled bias in beauty standards by introducing guidelines for inclusive AI-generated imagery.
Some effective trust-building practices include:
- Conducting regular bias audits, as seen in IBM's fairness framework
- Clearly communicating how AI influences marketing decisions
- Protecting data with encryption
- Offering users accessible options to manage their data preferences
The stakes are high - data bias alone can lead to up to 62% in lost revenue [18]. For companies, prioritizing ethical AI practices isn’t just about doing the right thing - it’s essential for long-term growth and customer loyalty.
Conclusion: Impact of AI Predictive Analytics
AI predictive analytics has reshaped how businesses approach marketing. A staggering 79% of marketing leaders believe AI will enhance productivity (50%), efficiency (45%), and creativity (38%) in their strategies [22]. These advancements are paving the way for a new era of marketing possibilities.
Companies experiencing faster growth are seeing a 40% boost in revenue through personalization [2]. This success stems from AI's ability to process massive amounts of customer data and provide actionable, real-time insights.
"AI is fundamentally reshaping marketing, offering more efficient, personalized and data-driven approaches to customer engagement." - Dr. Ismet Anitsal, Head of the Marketing Department at Missouri State University [20]
Take Netflix, for instance. Its AI-driven system creates over 2,000 "taste communities" to deliver hyper-personalized content recommendations. Similarly, airlines are using AI to adjust ticket prices dynamically based on real-time market trends [23].
AI doesn’t just improve personalization - it also drives real-time optimization and reduces costs. In fact, 84% of professionals agree that AI is essential for meeting modern customer expectations [21].
Area | Benefit | Outcome |
---|---|---|
Customer Engagement | Hyper-personalization | Faster response times |
Campaign Performance | Real-time optimization | Up to 50% reduction in ad spend |
Operational Efficiency | Automated analytics | 40% lower infrastructure costs |
With the global AI marketing market forecasted to hit $36 billion by 2024 [24], adopting these tools is no longer optional - it’s a necessity. Companies that integrate AI while maintaining ethical practices and transparency will position themselves as leaders in the digital age.
High-Growth Digital specializes in helping technical founders and engineering-driven companies leverage AI insights to turn complex products into market-ready strategies.
FAQs
How can businesses ensure their AI-driven predictive analytics are ethical and free from bias?
To ensure AI-powered predictive analytics remains fair and ethical, businesses need to prioritize transparency and accountability. This starts with openly documenting how data is gathered, processed, and utilized, giving stakeholders a clear view of how decisions are made. Regular audits of AI systems are equally important to uncover and address biases that might arise from historical data or flaws in the algorithms.
Another key step is assembling diverse teams to design and review AI models. Different perspectives can help identify ethical issues that might otherwise go unnoticed. Moreover, training AI on high-quality, representative datasets minimizes the risk of perpetuating existing biases. By taking these actions, companies can build trust and ensure their AI insights are fair and reliable.
How can companies successfully incorporate AI-powered predictive analytics into their marketing strategies?
To make the most of AI-powered predictive analytics in marketing, the first step is to pinpoint where it can have the biggest impact. This might mean focusing on areas like customer segmentation, lead scoring, or campaign optimization. By analyzing historical data, businesses can uncover patterns in customer behavior and preferences, which helps in crafting smarter strategies.
Once you've identified the focus areas, select AI tools that align with your marketing goals. It's equally important to ensure your team is well-equipped to use these tools effectively. Keep a close eye on how your strategies perform, using AI-driven insights to tweak and refine them. This ongoing process not only sharpens your approach but also boosts your return on investment (ROI) over time.
How does AI-powered predictive analytics enhance customer segmentation and personalization in marketing?
AI-Powered Predictive Analytics: Revolutionizing Customer Segmentation and Personalization
AI-powered predictive analytics is changing the way businesses understand and reach their customers. By processing massive amounts of data, AI identifies patterns and predicts customer behavior with impressive accuracy. Unlike older methods that mainly focused on demographics, AI dives deeper, using details like past purchases, browsing history, and real-time interactions to create behavior-based customer segments. This makes it possible to craft campaigns that feel tailor-made for individual needs.
But it doesn't stop there. AI also enables real-time personalization, adjusting marketing messages, offers, and content as customers interact with a brand. For instance, if someone browses a website or engages with an app, AI can quickly refine recommendations or promotions to match their actions. This not only keeps the content relevant but also boosts engagement and conversion rates. By delivering this level of personalization, businesses can build stronger relationships with their audience and see better results from their marketing efforts.